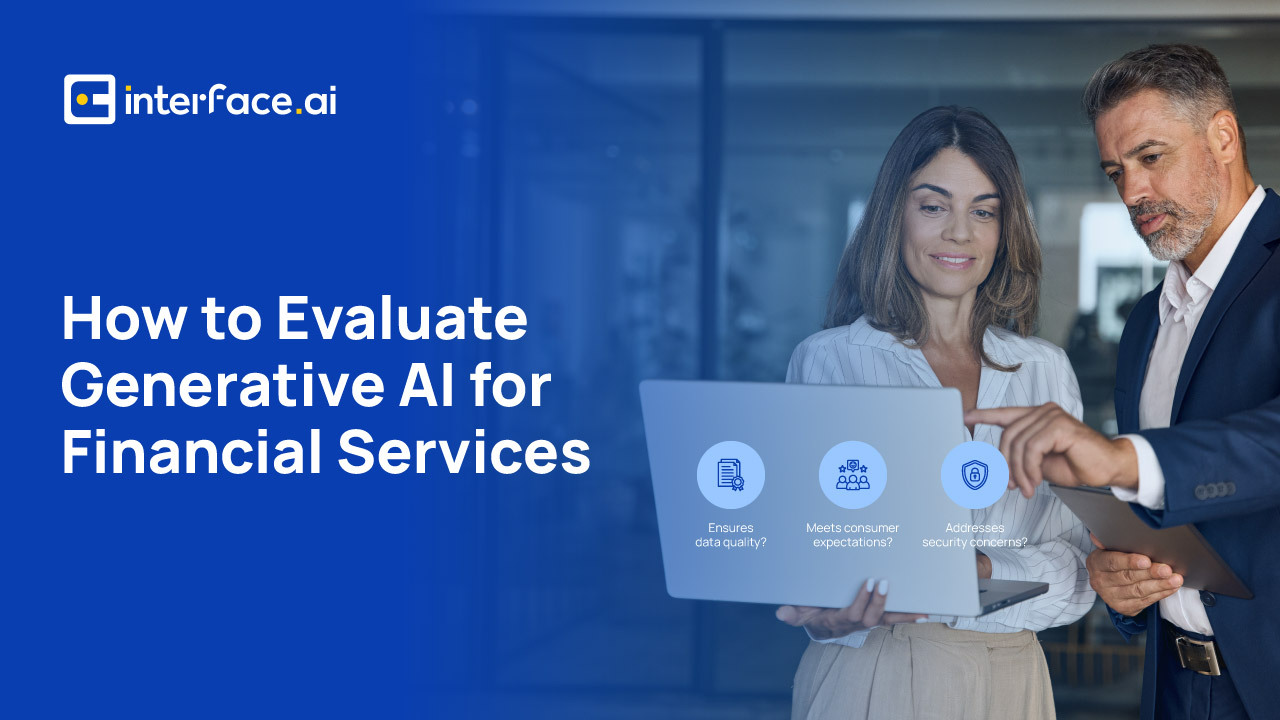
How to Evaluate Generative AI for Financial Services – 3 Key Questions to Ask
The promise of Generative AI in financial services is immense, but with this potential comes a new set of challenges that many are still learning to navigate.
There’s no doubt that Generative AI will establish itself as an indispensable tool in driving productivity, efficiency and personalization across organizations. As PWC has consistently predicted: “We see generative AI at scale not just as valuable, but also as inevitable.”
However, the road to widespread adoption in financial services will have its bumps. As vendors race to introduce GenAI solutions, some will build their own proprietary technologies, while others integrate third-party AI models for faster deployment. This rush to market will result in wide disparities in the quality, capabilities, and security of GenAI offerings, underscoring the challenges of Generative AI if not handled correctly.
This blog presents the three key questions every institution should ask Generative AI vendors to separate the wheat from the chaff, and the answers you should want to hear. You’ll learn the basics behind how to evaluate Generative AI models and be better equipped to distinguish between robust and weak offerings, ensuring you make the right Generative AI investment for your organization.
Question 1: What strategies & technologies do you employ to ensure data quality in your Generative AI solutions?
“Data quality makes or breaks Generative AI efforts” – Cornerstone Advisors
Data quality is the bedrock of successful Generative AI. Poor-quality or disorganized data can severely undermine the performance of AI systems, leading to inaccurate, unreliable, or even misleading outputs. This is because if the data fed into the GenAI system is missing information or contains errors, the AI will learn from these flawed inputs.
To understand a vendor’s data quality, you need to begin by evaluating Generative AI models. Poor data quality is most often the result of GenAI systems built on single LLMs. These GenAI offerings are trained on broad datasets to handle a variety of tasks for a variety of industries. They are built to be generalists rather than specialists. As a result, when these models are put to work in specialized domains like financial services, they usually struggle to meet the nuanced and domain-specific demands of banking. The results are even worse with open-source LLMs that solely rely on public internet data. While the internet offers a wealth of information, this “data landfill” is also cluttered with low-quality, irrelevant, or misleading data.
Data silos also undermine the performance of Generative AI systems. If data is stored in separate systems that don’t communicate with each other, the AI can’t access a holistic view of the information, leading to decisions based on partial or biased data.
GenAI models also often struggle with unstructured data. Unstructured data, like text documents, emails, or raw audio, poses challenges for Generative AI systems, which perform best with structured data – information organized in a clear format, such as databases or spreadsheets.
The right answer: Interconnected, domain-specific data lifecycle management
interface.ai addresses, and solves, these common Generative AI challenges with industry-leading data lifecycle management that ensures superior quality, accuracy, and relevance for financial services.
Firstly, interface.ai uses multiple Large Language Models (LLMs), rather than relying on a single LLM like many other AI providers. This is a crucial component of data quality and output and should be a key criteria of your Generative AI evaluation. This multi-LLM approach (known as a mixture of models) ensures each task is handled by the most suitable model, enhancing accuracy and relevance, and significantly reducing the risk of errors and “hallucinations” that are common with generalized models. Moreover, interface.ai’s LLMs are trained on domain-specific data so they can understand the complexities of financial services.
This domain-specific data is fused into interface.ai’s One Conversational AI Brain that powers its solutions. This unique data repository and processing engine aggregates data from over 1.5 million conversations every day, across every channel. This prevents data silos and fragmented intelligence that are common in channel-specific AI models. It also creates a collective intelligence that continuously learns from every interaction. This ongoing refinement process helps maintain data quality over time, ensuring that it remains accurate and effective as it encounters new information.
To mitigate the challenge of unstructured data, interface.ai uses a graph-grounded AI approach, in conjunction with its GenAI technology. This organizes unstructured data into structured knowledge graphs, enabling the GenAI to understand context, make more informed decisions, and reduce inaccuracies that often arise from relying on unstructured data alone.
To deliver even greater accuracy, interface.ai’s Generative AI assistants are also built on the organization’s pre-existing content. Users can easily upload knowledge resources – website URLs, documents, and videos – into the platform’s knowledge repository. The system then automatically scrapes, processes, and indexes all relevant information – and the GenAI assistant is ready to roll!
As well as improving accuracy, this also dramatically accelerates the bot training process. Most AI bots today are built manually by writing out each intent (the user’s goals) and the corresponding responses. This labor-intensive process can take months to complete, while interface.ai’s Generative AI assistants can be trained in minutes.
Question 2: How does your Generative AI meet today’s high consumer expectations?
“Customer confidence in self-service channels for transactional activities is leading them to expect similar outcomes for more involved requests.” – McKinsey & Co.
The shift to digital self-service channels, accelerated by the COVID-19 pandemic, has significantly reshaped consumer expectations. Self-serve began as a tool for quick, straightforward interactions, with bots able to manage simple information requests. However, as the usage of self-service has grown, so too has the complexity of the requests.
Today’s consumers expect self-service options to handle complex queries with the same efficiency and accuracy as simpler requests. This heightened expectation places considerable pressure on Generative AI (GenAI) systems to deliver reliable and sophisticated responses.
However, a major challenge in meeting these expectations is the issue of “hallucinations” – incorrect or nonsensical answers. As GenAI systems attempt to handle increasingly intricate queries, the risk of such errors becomes more pronounced. This erodes user trust and damages customer satisfaction. Make sure that your GenAI evaluation thoroughly explores this factor.
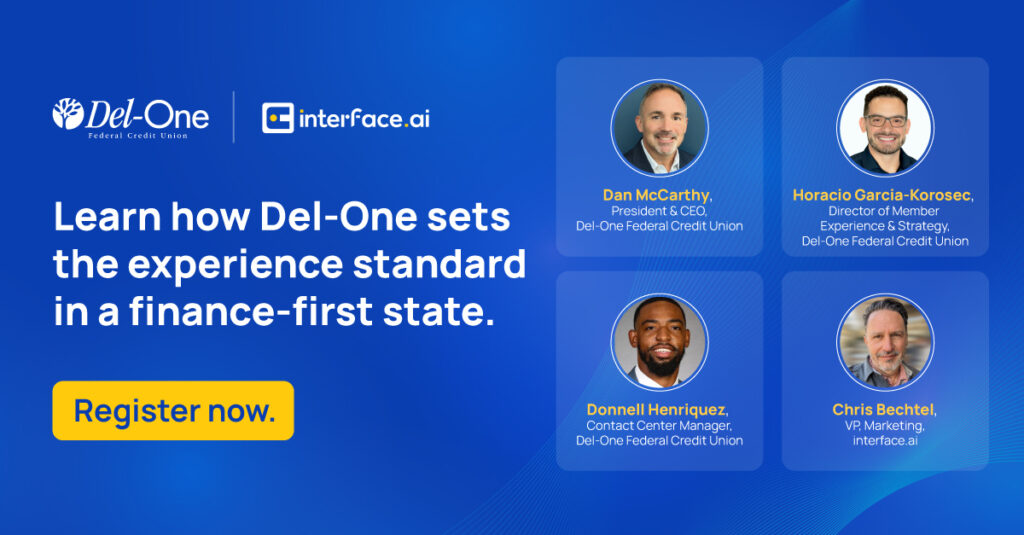
Exclusive Webinar: Creating a Great Member Experience in a Finance-First State: A Fireside Chat with Del-One FCU
The right answer: Deliver a GenAI solution that goes far beyond informational queries
interface.ai meets these elevated consumer expectations head-on with its advanced multi-domain LLMs and hyper-intelligent One Conversational AI Brain (see ‘Solution 1’ above). This sophisticated and unique architecture empowers its GenAI assistant to manage even the most complex financial queries with exceptional precision, surpassing the capabilities of all other financial services GenAI solutions.
The platform’s unique workflow system also transforms interface.ai’s Generative AI from an information retrieval system to a hyper-intelligent action-oriented assistant. Workflows are predefined, structured sequences guiding bot interactions. These workflows can override the standard Generative AI responses to power complex and action-orientated conversations that are more helpful to the user. They also give the organization greater control over the chatbot user experience during the most important conversations – another unique capability for financial service AI providers. You can also connect the workflows to core banking systems to resolve more queries and deliver true personalization.
Additionally, interface.ai incorporates a multi-layered feedback system that combines automated error correction with human-in-the-loop (HITL) review. This dual approach ensures errors are identified and corrected in real-time, while bringing in contextual understanding and ethical oversight from human review.
Question 3: How does our Generative AI address pressing security concerns and evolving regulations?
“Trust in AI is key to realizing value from this technology. But many companies struggle to overcome the perceived risks associated with it.” – Accenture
When anyone considers what challenges Generative AI faces, security and privacy always top the list. The integration of Generative AI into financial services has introduced a host of security and privacy challenges that organizations must navigate carefully. One of the most pressing concerns is the risk of data breaches and unauthorized access. Generative AI systems process and store vast amounts of sensitive information, making them prime targets for cyberattacks.
Regulatory compliance adds another layer of complexity for financial institutions. Banks and credit unions must adhere to strict data protection laws and AI ethics standards, which are constantly being updated to keep pace with technological advancements. Responsible AI has gained steam within this landscape, calling for the safe and ethical deployment of AI. The potential for bias, harm, and the generation of unreliable information poses more challenges for Generative AI and financial institutions.
The right answer: Generative AI founded on Responsible AI principles
Since 2015, interface.ai has been at the forefront of AI in financial services, introducing the industry’s first fully-functioning Generative AI assistant. Central to this pioneering approach is the development of Responsible AI. The platform’s architecture is not just built to be powerful, but also to be secure, ethical, and accountable.
Interface.ai ensures that its Generative AI solutions meet the stringent demands of the financial services industry through several approaches, guardrails, and compliances. Here are just a few of these unique approaches:
- Multi-Layered Authentication: interface.ai is the only AI provider for financial services that delivers the most secure trifecta of risk-based authentication – AI, biometrics and caller ID. This unique, multi-layered defense system effectively verifies user identities and significantly reduces the risk of data breaches, offering a higher level of security than all other standard financial service AI solutions.
- Integration Layer and Secure Processing: The platform features an integration layer that acts as a secure gateway between the Generative AI system and core banking systems. This layer ensures that only authorized, validated requests can access critical financial data, providing an additional layer of protection.
- Auditable Processing Engine: Interface.ai’s auditable processing engine meticulously records every action and decision made by the AI. This includes detailed logs of the reasoning process, data inputs, and interpretation methods. This transparency not only ensures compliance with regulatory requirements, but also supports accountability and ethical oversight.
Read more about how interface.ai’s AI solutions are built to deploy Responsible AI for financial services.
Wrap-up
Thorough GenAI evaluation is critical. As Generative AI continues to shape the future of financial services, it’s crucial that organizations choose the right AI vendor to support them in this journey. AI, and especially Generative AI is not built equally.
interface.ai is the leader in AI for financial services. With purpose-built AI for banks and credit unions, enhanced by the industry’ only proprietary Generative AI in use today, interface.ai is the perfect partner for banks and credit unions wanting to take advantage of what AI has to offer.
To learn more about how interface.ai can help your organization and evaluate our Generative AI assistants, click here.
Discover the Latest Insights on Interactive Intelligence for Banking Newsletter
Join the newsletter to receive the latest updates in your inbox.